STAT6077 Key Topics in Social Science: Measurement and Data
Semester 1, 2023/2024
Assignment: STAT6077 Key Topics in Social Science: Measurement and Data
Administrative Arrangements
This assignment contributes 100% of your overall mark for STAT6077. The deadline is 4:00pm on Monday 8th January 2024 (Week 15).
Electronic Coursework Submission
You should submit coursework electronically via the TurnitinUK plagiarism device on Blackboard, by not later than the published date and time. Turnitin is a plagiarism detection tool which checks your work against electronic sources and other submissions for the same assignment.
Login to the Blackboard site for this module and select the Assignments link from the left-hand menu. Find the coursework and click View/Complete. There will be a series of screens to complete and then you will upload your assessment as an electronic file.
For a tutorial explaining the submission procedure in detail please go to the iSolutions website: https://elearn.soton.ac.uk/article-categories/tii-student/
Once you have submitted your assignment through Turnitin, you are able to download a copy of your Digital Receipt. It is recommended you do this as soon as your submission is complete and keep a copy, since it may be required as proof that you have submitted your work. When your submission is successful, you are taken back to the Assignment Dashboard and a message is shown that says “Submission uploaded successfully Download digital receipt”. You can select “Download Digital Receipt” to download your receipt. If you come back to the dashboard in the future, the message will not be shown instead you can use the icon to the far right of the submission item to download a copy of your Digital Receipt. If you do not receive a submission ID number and cannot download a Digital Receipt, it means that you have not submitted. If this is the case, you will be penalised. If you think you have submitted but cannot obtain a Digital Receipt, then you should contact the module coordinator as soon as possible.
You are advised to leave plenty of time before the deadline for electronic coursework submission. Delays due to computer ‘glitches’ will not be considered as justification for late submission.
Penalty for late submission
When coursework is set a due date for submission will be specified and there will be associated penalties for handing in work late unless a deadline extension has been formally granted. Work submitted up to 5 days after the deadline will be marked as usual, including moderation or second marking, and feedback prepared and given to the student. The final agreed mark is then reduced by the factors in the following table.
University Working Days late |
Mark |
1 |
(final agreed mark) * 0.9 |
2 |
(final agreed mark) * 0.8 |
3 |
(final agreed mark) * 0.7 |
4 |
(final agreed mark) * 0.6 |
5 |
(final agreed mark) * 0.5 |
More than 5 |
Zero |
For example, if your mark for the coursework is 63% but you hand in your work 3 working days late, then your final mark would be 63*0.7 = 44.1%.
Troubleshooting and Academic Integrity
This is not group work. Your report must be your own work and based on your own analysis. You are not permitted to show any student your written work or computer code/syntax or outputs. Copying includes using another student’s computer code/syntax, output or graphics. Turnitin submissions will be examined, and if academic integrity is deemed to have been breached, there are a range of penalties that may be applied. Your attention is also drawn to the section regarding academic integrity in the Module Outline.
Students for whom English is not their first language sometimes feel it is easier to write in their home language and then translate their work, sometimes using translation software. We encourage you to work in English throughout. We have some evidence that using translation software may lead to the translated text being identified as plagiarism, as the software reverts to common phrases. The University does not recommend or endorse companies offering proof-reading or translation services.
The Tasks
Answer the research questions outlined in Tasks 1 to 4. Please also provide the complete Stata do-files for Tasks 1, 2 and 4 in the appendix of your assignment since they will be evaluated together with the results of the sub-questions in each task. Please do this by copying the text from your do-file into the appendix of your assignment, rather than by attaching the do-file as a separate file. Please provide Stata results relevant for answering the questions in the main text of your assignment.
TASK 1: POVERTY AND INEQUALITY
In this task you will use data from the 2006/07 Family Resources Survey (FRS_data_0607.dta). The data contain observations from 55,778 individuals (adults and children) living in 24,199 households in the UK. Note that, since weights are not included in the dataset, you do not need to use weights for this task. The table below provides a list of variables.
Analysis of FRS data (Note: please provide your Stata do-file in the Appendix.)
Variable name |
Description |
sernum |
Unique household identification number |
indinc |
Individual income gross |
nindinc |
Individual income net |
age80 |
Age (all adults aged 80 over are coded as 80) |
famsize |
Number of individuals in household |
empstati |
ILO employment status variable |
By analysing this dataset, answer the following questions:
a) How does the choice of gross or net household income influence our estimate of the percentage of the population that are ‘poor’? To answer this question, calculate the percentage poor for gross and net household income separately and then compare the results. For each income type, use three relative poverty lines: 40%, 50% and 60% of the median household income. [14]
b) How does the composition of the poor (focusing on children and the elderly) vary according to the choice of equivalence scale? To answer this question, compare the percentage of children (defined as under 18) and the percentage of the elderly (defined those aged 65 and older) among the poor. Use net household income, and a relative poverty line that is 60% of the median. Use three different equivalence scales of your choice. [8]
c) Explain any assumptions that you are making in your analysis for a) and b). [3]
[TASK 1 total: 25]
TASK 2: EDUCATION
Task 2 asks you to analyse educational achievement data from PISA.
Analysis of PISA data (Note: please provide your Stata do-file in the Appendix.)
Your task is to examine the educational achievement of 15 year olds in Denmark. The data set is called “PISA_2012_DEN”, a reduced version of the PISA 2012 data set. See the following table for a description of the variables in the data:
Variable name |
Description |
country |
=DEN for Denmark |
stidstd |
Unique student identifier |
schoolid |
Unique school identifier and Primary Sampling Unit |
w_fstuwt |
Student weight variable – please use for all the calculations |
math |
PISA achievement score (mean of the 5 plausible values) – this is your dependent variable |
sex |
Sex: 1= female, 2=male |
hisei |
Ganzeboom index, continuous variable. Higher values indicate higher parental socio-economic status |
hisced |
Highest educational level of parents ( 2-ISCED 2 or lower; 3-ISCED 3b,c; 4-ISCED 3a,4; 5-ISCED 5b; 6-ISCED 5a,6) |
immig |
Immigration status (1: native; 2: second generation migrant; 3: first generation migrant) |
fjob |
The current job status of the father of the student: 1=Full-time, 2=part-time; 3:=Not working, but looking for a job; 4= Other (inc. stay-at-home) |
a) Estimate the population mean and population standard deviation of math and of hisei. [2]
b) Use regression to describe the relationship between PISA math achievement and three variables: hisei, hisced and immig
· First consider each of these three variables separately, in three different regression models.
· Then, in a fourth regression model, include each of these three variables together in the same model.
Present the results of the four models in one single table that includes all important information in a way that results of the regressions can be easily compared. [6]
c) Interpret the results from your models in b). [8]
[TASK 2 total: 16]
TASK 3: SOCIAL MOBILITY
Parts a)-c) do not require you to conduct your own data analysis but ask you to interpret existing analysis of BCS data; part d) asks you to reflect on the literature on social mobility.
Interpretation of British Cohort Study analysis
Here we examine social mobility, based on existing analysis of the UK British Cohort Study (BCS; 1970) data set. The following page contains a log of Stata output from analysis of these BCS data. The table below contains a description of the relevant variables.
Variable name |
Description |
bcsid |
BCS id number |
b34soc |
Social Class: Individual’s Job at Age 34 (1- unskilled; 2-partly skilled; 3-skilled manual; 4-skilled non-manual; 5-managerial/technical; 6- professional). |
bsex |
Individual’s gender: 1-male; 2-female |
b10fsoc |
Social Class: Occupation of Father when individual aged 10 (1- unskilled; 2-partly skilled; 3-skilled manual; 4-skilled non-manual; 5-managerial/technical; 6- professional). |
b34hq5 |
Highest academic qualification at age 34 (0-none; 1–cse; 2–gce o level/gcse; 3-a level/ssce/a-s level; 4-degree/ dip. h.ed; 5-higher degree/pgce) |
Answer the following questions.
a) Interpret the results of the analysis on the following page. [6]
b) Describe, using no more than 150 words, the quality of this empirical evidence as an assessment of social mobility in the UK. [3]
c) How would you use this dataset to assess whether social mobility in the UK varies by an individual’s gender? [3]
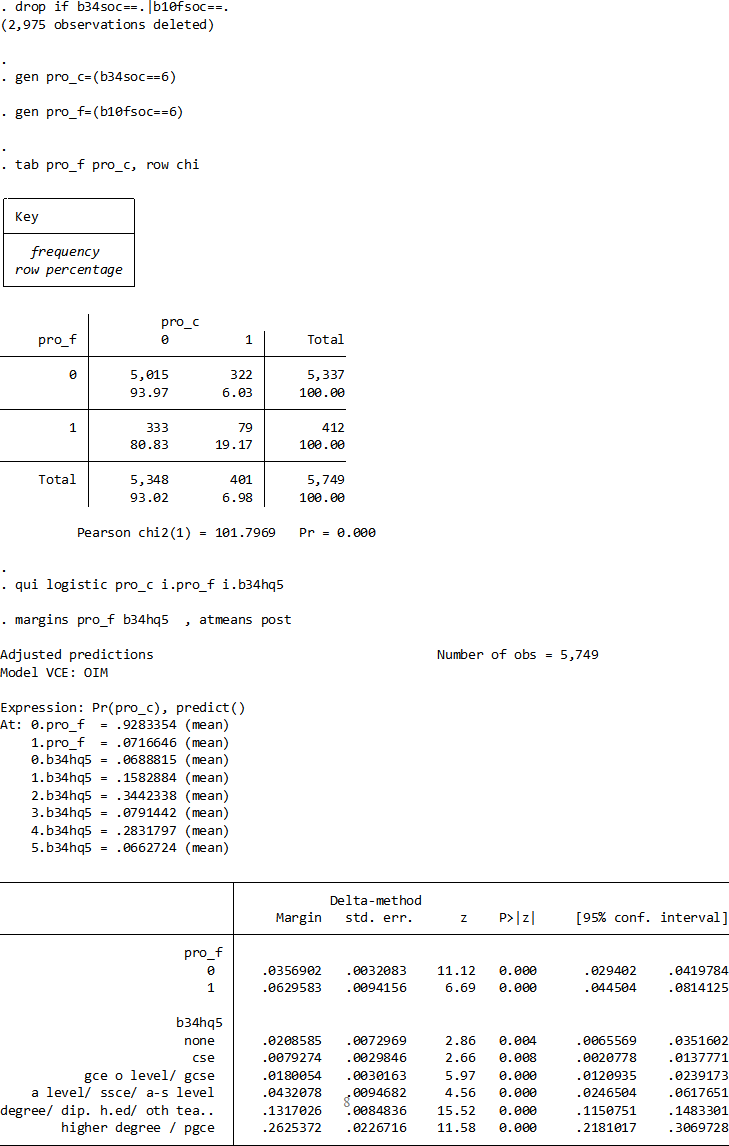
d) ‘Social mobility in the UK is in decline’. Discuss this statement, drawing on relevant academic research.
[Note: 350 words maximum for this answer. This word total does not include the list of references]. [12]
[Task 3 total: 24]
Task 4: EMPLOYMENT
For Task 4, parts a)-e) relate to the analysis of LFS data; part f) does not involve data analysis. Before answering questions a)-d) you will need to define a ‘working household’ variable.
Analysis of LFS data (Note: please provide your Stata do-file in the Appendix.)
You will examine what factors are associated with the probability of an individual being in a working household. The dataset LFS_data_2018 is a shortened version of the 2018 UK Labour Force Survey and includes the following variables:
Variable name |
Description |
hserialp |
Household identifier |
casenop |
Case number |
pwt18 |
Person weight |
sexx |
Gender of respondent |
age |
Age of respondent |
ilodefr |
Economic activity |
regionx |
Region |
hiqul15d |
Highest educational qualification (detailed grouping) |
fbx |
Place of birth (whether born outside the UK) |
ethukeul |
Ethnicity |
bhealthx |
Bad health that limits paid work |
Note that:
· Each row in the data corresponds to an individual (casenop). Remember that the Labour Force Survey is a household survey, containing information on all individuals in the households that were interviewed. Therefore, in our data, each individual is also nested within a household (identified by hserialp).
· The data contain individuals aged 16-65 inclusive.
· The person weight, which adjusts for nonresponse, is given by the variable pwt18.
· For the purposes of this analysis, there is no need to account for clustering within households.
Definitions: For the purposes of this analysis:
• ‘working age’ is defined as aged between 18-60 inclusive (for both men and women).
· a ‘working household’ is a household that:
o contains at least one person aged 18-60 inclusive (i.e. of ‘working age’), and
o in which every household member of ‘working age’ is in employment.
Questions
a) What proportion of people aged 16-65 are of working age? What proportion of people aged 16-65 are in working households? [3]
b) Examine, using cross-tabulations, (i) regional differences in highest educational qualification; and (ii) regional differences in bad health. Provide the tables and interpret the results. [5]
c) Use regression to describe the relationship between the probability of an individual being in a working household and three variables: regionx, hiqul15d and bhealthx.
· First consider each of these three variables separately, in three different regression models.
· Then, in a fourth regression model, include each of these three variables together in the same model.
Report the results of the models in terms of predicted probabilities. Present the results of the four models in one single table that includes all important information in a way that results of the regressions can be easily compared. [8]
d) Interpret the results of your regression analysis in c). [8]
e) Use logistic regression to investigate how the probability of an individual being economically active varies according to their ethnicity and sex. Present and interpret your results. [6]
f) Explain how you might expect the relationship between two different UK measures of unemployment - based on (i) the ILO definition and (ii) a count of unemployment benefit recipients - to change over the economic cycle. [5]
[Task 4 total: 35]